Clinical Minds Blog
Gain exclusive clinical trial insights and learn how Medidata’s offerings are solving the latest industry challenges.
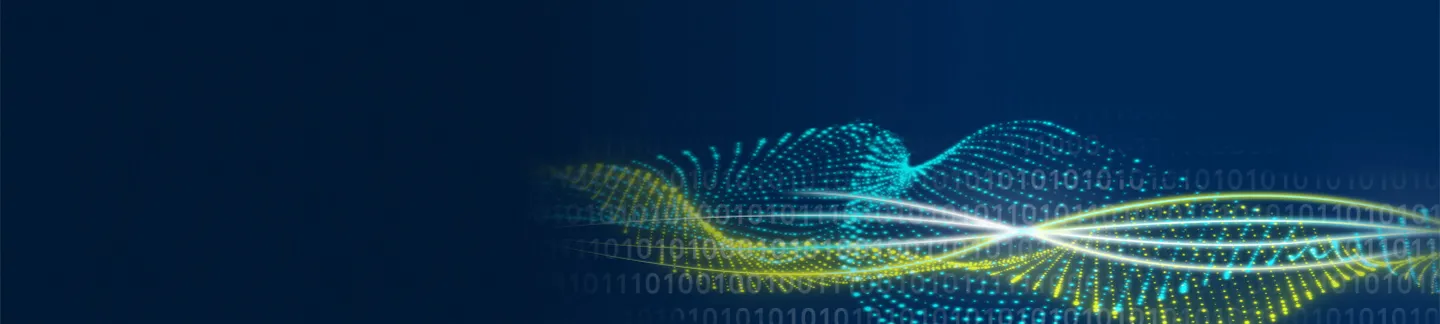
Contact Us
Ready to transform your clinical trials? Get in touch with us today to get started.
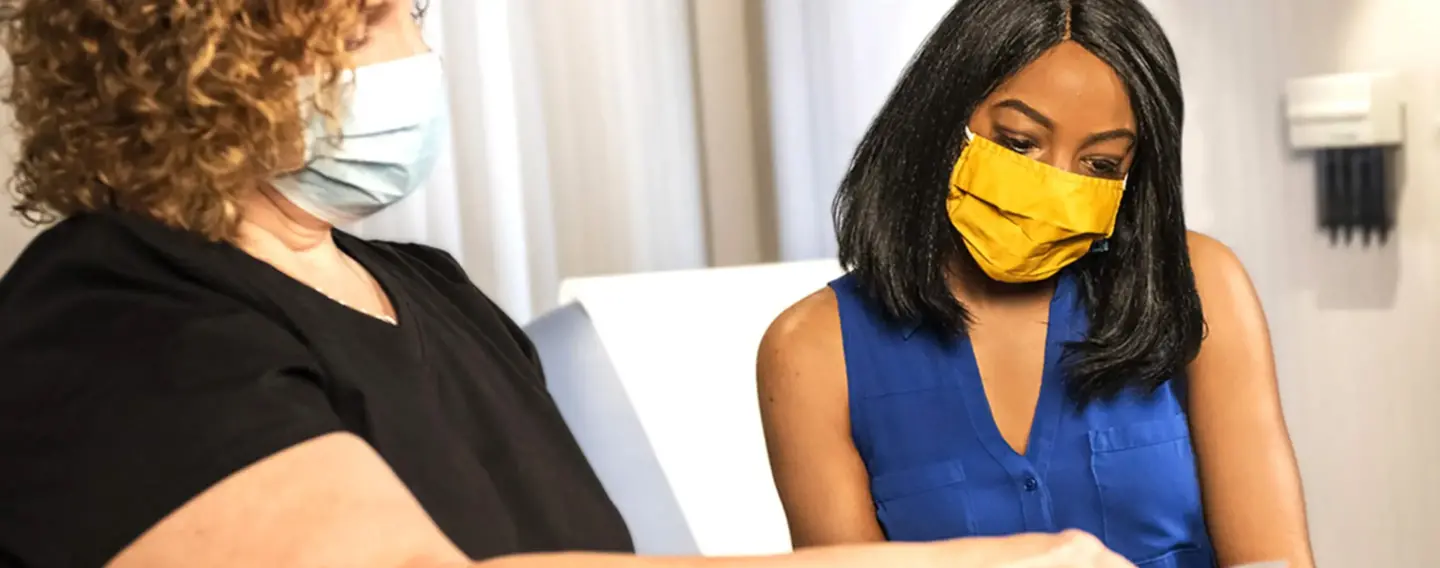
Subscribe to Our Blog
Receive the latest insights on clinical innovation, healthcare technology, and more.