Modernizing Clinical Data Capture and Management
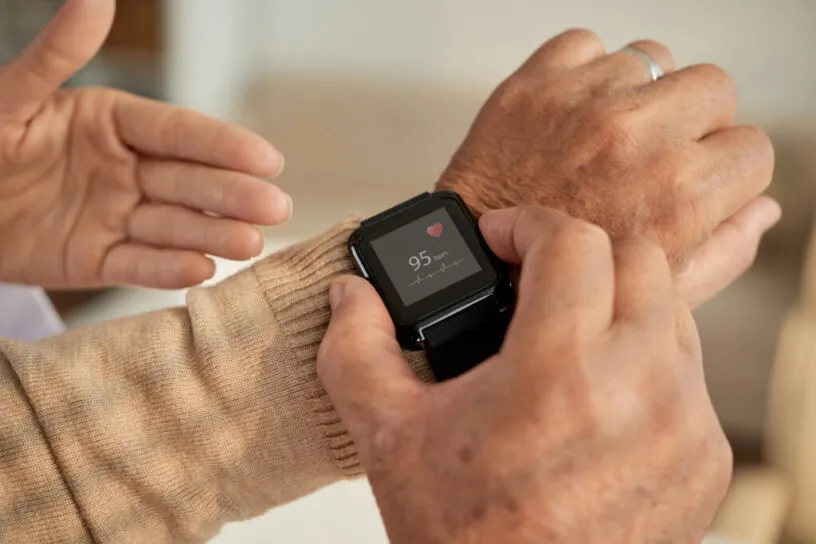
Clinical trials are becoming more complex and a confluence of forces—including greater emphasis on patient experience, increasing protocol complexity, and precise value differentiation—is accelerating this complexity. Trials have evolved to include an ever-expanding array of data sources, increased data volume and precision, decentralized clinical trials (DCT), and adaptive designs.
These environmental and industry changes have led to significant clinical data capture and clinical data management challenges because clinical data management (CDM)—which includes how data is reviewed, cleaned, and locked—technologies and processes have not progressed at the same breakneck speed. Additionally, technology ecosystems are often built with a mix of disparate tools that are homegrown or from different vendors, and the individual components lack interoperability. Consequently, clinical trial data management is done with tools and processes that are not able to evolve and adapt at the speed required to support the realities of the ever-changing modern clinical trial. Furthermore, since data first began to be electronically collected using eCRFs (electronic Case Report Forms), the data acquisition landscape has evolved greatly, as depicted below.
The time for advancement in clinical data management is now. It is currently being modernized by unifying data and workflows on a cloud-based platform. This is transforming clinical data management to be scalable, flexible, intelligent, and interoperable with a variety of third-party systems so that it can sustain drug development into the future. That future includes more decentralized study designs where visits are monitored remotely, drugs are shipped to patients, and data is captured directly from patients regardless of location.
By leveraging a unified clinical data management platform, sponsors and CROs can achieve greater efficiencies that result in quicker study closeouts, automated data standardization, and the development of comprehensive views of patient data that are not easily achieved using traditional clinical data capture and management processes.
For instance, by using scalable and intelligent risk-based clinical data management (RBDM) processes, proactive and continuous virtual oversight of data quality happens in almost real-time. This can significantly reduce edit checks and automate the majority of data reviews. This type of risk-based approach can dramatically shorten the time to database lock, which can now be achieved in a matter of hours or days instead of multiple weeks.
Modernized Clinical Data Management platforms also need to intelligently automate the transformation of data for analyses.
Highly comprehensive views of patient data can also be developed. Such comprehensive profiles provide a rare window into how patients are really affected by drugs and disease. Modernized CDM platforms merge different data streams—including both subjective (e.g., PROs) and objective data (e.g., wearables/sensors)—through a common model so that you can easily and quickly develop unified 360° views of patient data that are hugely informative. They also reduce the risk to data integrity, quality, and patient safety. The importance of gaining deep patient insights is very important in today’s environment where stakeholders across the healthcare enterprise—regulators, payers, and patients—are placing greater emphasis on patient-centricity and value demonstration of new therapies.
On the road to modernizing clinical data management, a holistic aggregation of data is required. This means that a complete study and its patient dataset have the context of the whole study design. This requires intelligent and automated tools and workflows and a user experience that can operate on the complete study and patient dataset agnostic to its source. Examples of these intelligent, automated tools and workflows include the following:
- Centralized study design and data
- Auditable, permission-driven issue management capability on all of your data
- “Analytics first” approach to drive and direct users to action
- Intelligent approach to data review
- Data source-driven workflows and activities
- Predictive approach to resource allocation
Modern CDM platforms provide the necessary framework needed to achieve the speed and scale required of modern trial designs including:
- Faster database locks,
- Submission ready-outputs, and
- Comprehensive views of patient data.
Download our white paper to learn how to succeed in an increasingly complex clinical trial world:
Contact Us
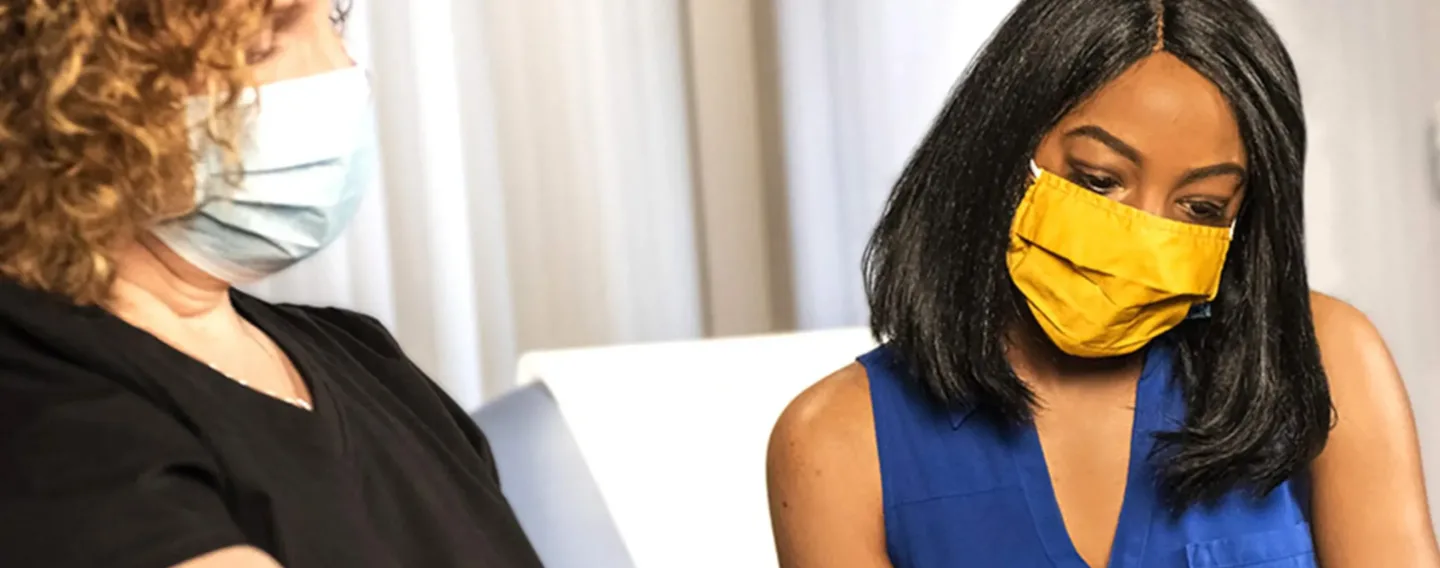