Optimizing Your Endpoint Adjudication Process Today and Beyond
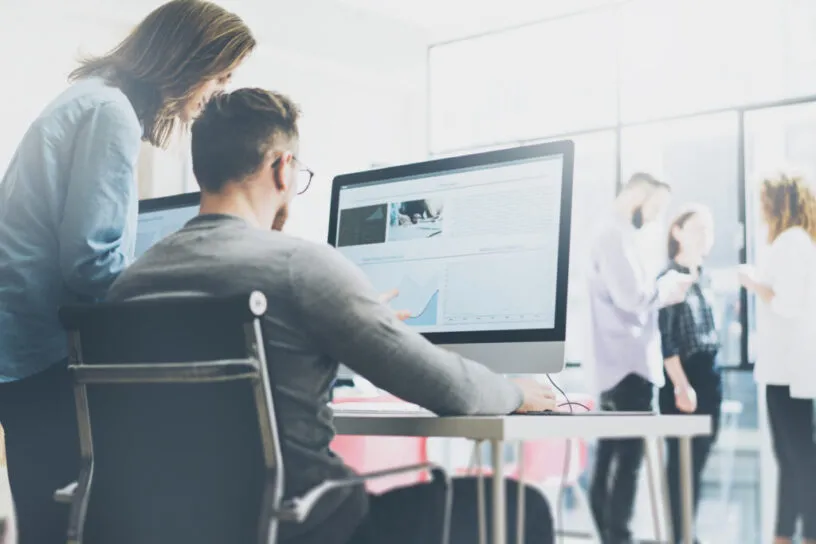
Medidata recently hosted a webinar titled Optimizing Your Endpoint Adjudication Process Today and Beyond. The event brought together industry leaders to discuss the trends and insights in modernizing the endpoint adjudication process.
Speakers included:
- Andreas Järemo - Product Director, Digital Health, AstraZeneca
- Syed Hussain - Medical Director of Safety Services, Baim Institute for Clinical Research
- James Riddle – Vice President, Research Services, Advarra
- Andrea Falkoff - Senior Director, Product Management, Medidata
The panel discussed the benefits and challenges of introducing automation into the endpoint adjudication process, including what roles artificial intelligence/machine learning (AI/ML) and natural language processing (NLP) may play and the potential impact of automation on cost, time, quality, and compliance.
Overall, the decision to automate processes in endpoint adjudication depends on multiple factors such as trial size, trial complexity, stakeholder integration, and the potential benefits of efficiency and data management. While the potential benefits of automation, AI, ML, and NLP in the adjudication process are recognized, these technologies must be implemented carefully, with validation and human oversight to make sure they’re accurate, reliable, and remain compliant with relevant regulations, such as 21 CFR Part 11 and EMA Annex 11.
Read on for a summary of the main questions discussed by the panel. This information offers key insights for those considering optimizing their endpoint adjudication process.
Is it time to dump the spreadsheets? When is the right time to automate endpoint adjudication?
Spreadsheets may continue to be used for sporadic, small studies where a small number of events are expected. But when it comes to larger studies or many small studies, spreadsheets lack scalability and are highly prone to manual data entry errors. They’re easy to manipulate and need more version control.
Automating the endpoint adjudication process for studies with data housed outside of spreadsheets offers numerous benefits, including improved efficiency and enhanced data quality. But the right time to automate depends on various factors, such as the size and complexity of the study, the volume of data to be managed, and the available resources for implementation. Organizations should thoroughly evaluate their specific needs and outline the potential for automation to significantly streamline processes and improve overall efficiencies.
The panel emphasized that each clinical trial adjudication project is unique and automation levels vary widely among sponsors, CROs, and sites—with many still relying on hard-copy records and spreadsheets. Initiating conversations with experienced professionals with in-depth expertise in automating specific endpoint adjudication processes is an essential first step.
What does automation mean? Which processes can we automate?
Automation in the context of endpoint adjudication covers a broad spectrum of possibilities. It can detect and remove protected health information (PHI), facilitate parallel adjudications, assign discordant cases to different adjudicators, and extract relevant information for medical monitor reviews. Implementing ML techniques can help adjudicators make consistent decisions by learning from past conclusions and alerting adjudicators to similar cases.
By automating and validating processes per regulatory guidelines, organizations can prevent or minimize human errors, reduce the time and effort needed for manual tasks, improve data quality and integrity, and ensure compliance with regulatory requirements.
How does AI/ML fit into endpoint adjudication? What does it mean? Is it all hype? What about NLP?
The speakers recognized the expanding role of AI/ML in the adjudication process, but they stressed that these technologies are decision-support tools that still require the ongoing involvement of humans. These algorithms do not make final decisions but are designed to enhance productivity by highlighting patterns, trends, and potential risks across complex datasets. The algorithms can help adjudicators make consistent decisions, and the performance of the algorithms is being continuously improved by having humans assess the accuracy of the findings and providing feedback to the algorithm.
On the other hand, NLP facilitates the understanding and processing of human language by computers, enabling extracting relevant information from unstructured clinical trial documents and streamlining data analysis. NLP is primarily used to extract information for adjudication, with human judgment still involved before submission to the adjudicator. But ensuring compliance and building trust in NLP-based processing can be a challenging journey, and users should be aware of the strengths and weaknesses. These technologies are evolving rapidly, and they have already demonstrated their ability to enhance efficiency and accuracy across the clinical research spectrum.
What are the regulatory considerations?
Compliance with relevant regulatory guidelines and standards, such as those established by the FDA or EMA, remains of utmost importance in optimizing the endpoint adjudication process. Compliance with regulations like 21 CFR part 11 and EMA Annex 11, which govern electronic records, remains essential to validate the trustworthiness of this information. Validation of automated processes is also necessary to make sure they are functioning as intended. Data integrity, privacy, and security should be taken into consideration. Global trials need to consider the specific regulations of different countries, such as HIPAA (Health Insurance Portability and Accountability Act) in the US and the General Data Protection Regulation (GDPR) in the EU.
It’s advisable to have frequent and open communications with regulatory authorities to make sure the implemented processes and technologies meet the required standards and guidelines, ultimately leading to reliable and trustworthy endpoint adjudication outcomes.
What are the impacts on cost, time, and quality?
The introduction of automation in the endpoint adjudication process yields cost, time, and quality benefits. It reduces manual efforts, streamlines workflows, and eliminates error-prone spreadsheets, leading to cost savings through improved operational efficiency. Automation can expedite the adjudication process, shortening time-to-decision and enabling faster clinical trial timelines.
Automation also enhances the quality of endpoint adjudication by minimizing human errors, ensuring consistency, and promoting standardized processes. Leveraging AI/ML algorithms let organizations leverage advanced data analytics and pattern recognition, resulting in more accurate and efficient identification of relevant endpoint data. This, in turn, improves data quality, reduces discrepancies, and enhances the overall reliability of endpoint adjudication outcomes, ultimately contributing to well-informed decisions.
Summary
The webinar panel discussed the benefits and challenges of automating the endpoint adjudication process, highlighting the roles of AI/ML and NLP and the potential impacts on cost, time, quality, and compliance. The decision to automate depends on various factors, and it was emphasized that automation should be implemented carefully to ensure accuracy, reliability, and compliance with regulations. Conversations with experienced professionals in automating endpoint adjudication processes are recommended.
The panel also emphasized that automation could bring numerous benefits to the adjudication process, including time and cost savings and improved quality. Still, its implementation requires careful planning, validation, and consideration of the specific needs and resources of each study.
Watch the full recording of the panel discussion to hear more.
Ready to optimize your endpoint adjudication process? Discover Medidata Adjudicate.
Contact Us
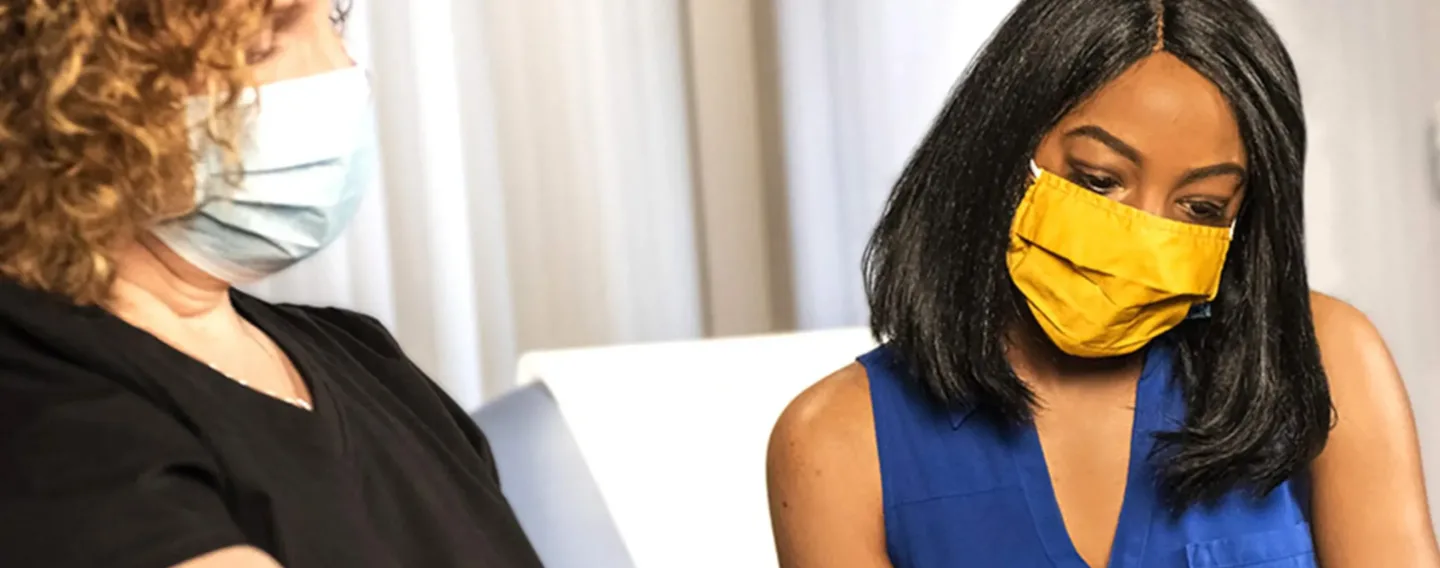