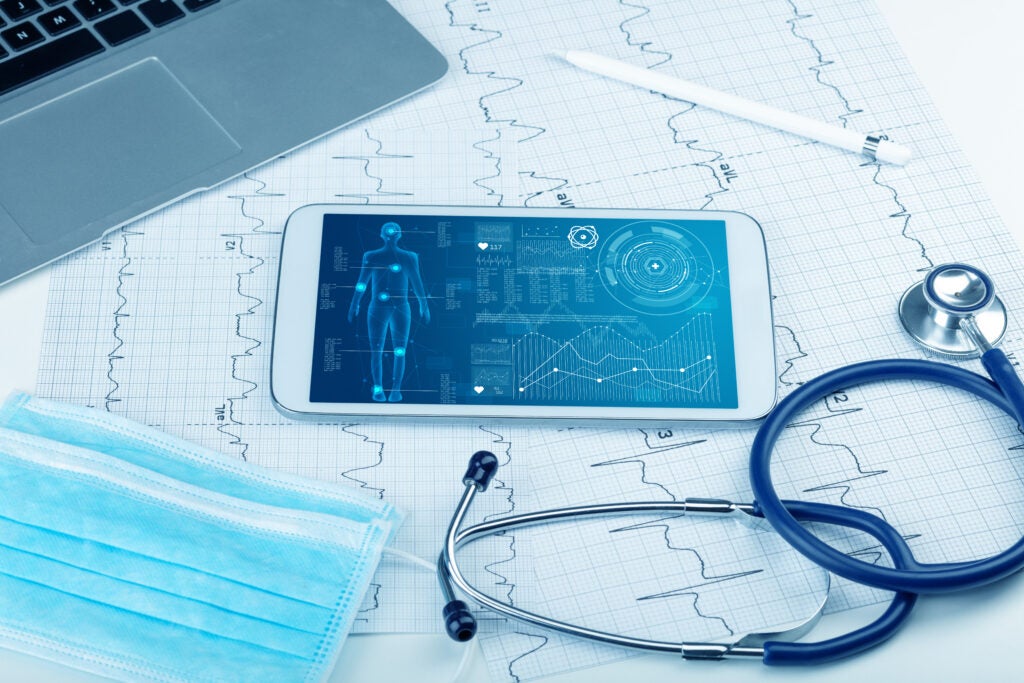
The use of generative (gen) AI in clinical trials has the potential to decrease the generally lengthy timelines and high costs (Boston Consulting Group, 2023). While it’s been a challenge to gain a full understanding of the implications and capabilities of gen AI—especially in the healthcare and life sciences industry—one promising area is in protocol design.
Protocol design is defined by a comprehensive plan that outlines study objectives, eligibility criteria, treatment interventions, and data collection methods to ensure the safe and ethical conduct of a clinical trial, while generating reliable results for evaluating the safety and efficacy of the investigational therapy.
The process of designing a clinical trial protocol can take months to years and is prone to delays or amendments after the trial has started. These delays may happen for a number of reasons, including safety concerns, changing regulatory requirements, or logistics not going as planned. Protocol amendments are highly disruptive and are generally the cause of time delays and unbudgeted costs (Tufts University, 2023). Historical clinical trial data can improve a clinical trial protocol by informing decision-making based on past trial successes or failures, and limiting causes for protocol amendments and delays—ultimately increasing the probability of technical and regulatory success.
But most organizations don’t have access to cross-sponsor clinical trial data because it contains both sensitive patient information and protected intellectual property, and remains siloed within organizations. With generative AI, organizations can now leverage synthetic historical clinical trial data. Synthetic data can be created from historical clinical trial data by using algorithms to generate new datasets that retain the key and nuanced statistical properties of the original data, such as distribution patterns and correlations between variables, but doesn’t contain any actual information from individuals or events in the real world.
Optimizing clinical trial protocols with synthetic data powered by gen AI can allow for more specific study parameters and help reduce site and patient burden. Generative AI has the ability to take in vast, complex, and highly sensitive data and information in short periods of time—in this case from existing trial protocols and data from historical clinical trials, to then be used to make more informed decisions that result in safer, more effective study protocols. This can save research and development teams great amounts of time—which accelerates the pace of clinical innovation and saves money.
These algorithms ingest data from past clinical trials to inform insights for new study protocol designs and run trial scenario modeling. These insights then inform key decisions, including ideal sample sizes, or identifying optimal inclusion/exclusion criteria.
This criteria helps limit confounding variables and ensure participant safety. Synthetic trial data can also provide insight on appropriate duration of a study, optimal site locations, and metrics to record. These are tasks that often take up more time than anticipated, and if selected incorrectly, cause significant delays in a trial. Insights provided by gen AI have the potential to lead to more effective and complete clinical trial protocol designs.
Medidata AI Simulants generates synthetic data from cross-sponsor historical clinical trial data and ensures a high fidelity dataset. Because of this highly robust set of data, sponsors have the ability to leverage Simulants to analyze existing data and derive insights to improve protocol design with better decision-making, ultimately increasing the efficiency of the trial and the probability of technical and regulatory success.
It’s crucial that we work in harmony with AI to ensure timely, yet safe clinical trials. Generative AI has shown to be helpful in the labor intensive, data analytics and ingestion tasks; with Simulants specifically, it can do so while protecting patient privacy, given the nature of synthetic datasets.
Generative AI can enhance many aspects of the drug development life cycle, including protocol design. Leveraging algorithms and existing data, gen AI solutions can learn from past protocols and provide crucial insights for an efficient and robust protocol design. including more accurate patient cohorts and effective endpoints. Using generative AI to derive better insights can greatly reduce decision-making and planning.
Learn more about Medidata AI Simulants.