Patient Centricity Needs Data Centricity
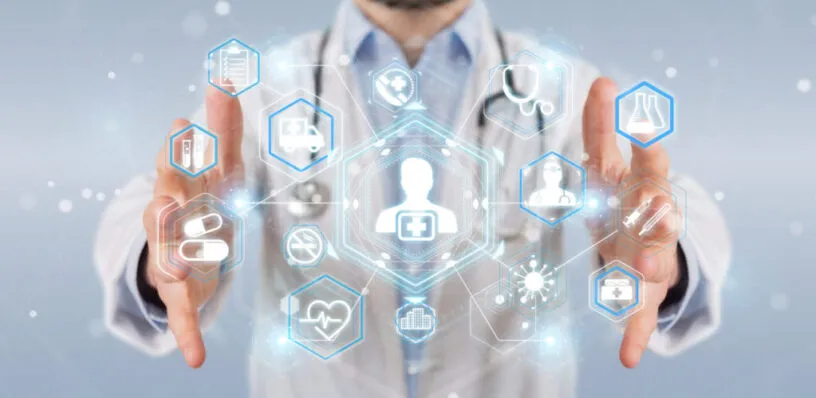
This blog was authored by Wayne Walker, SVP, Rave Platform Technology at Medidata.
Patient centricity and patient retention go hand in hand; both are critical to a successful clinical trial. The best way to achieve both is to enable patients to participate in clinical trials in a way that best suits their preferences, behavior, and needs.
Depending on the individual, they may participate in a study through face-to-face interactions because of the personal care and attention received through a site visit. Other patients may want to reduce the need for in-person appointments—particularly if the site is far from where they live or if their condition makes it difficult to travel. They may prefer to use an app to complete a diary and questionnaires. Others may be happy to use sensor technologies that offer continual monitoring. Depending on the study, a mixture of these methods may be required or preferred—even for a single patient at different stages of a study.
With all the resources at hand as an industry, there’s never been a better opportunity to provide clinical trial access to a broader, more diverse patient population—delivering more flexible methods that let patients choose how they participate in a trial while enabling more accurate insights into the patient’s condition and study experience.
But there’s so many disparate data acquisition systems available to sponsors, including EDC (electronic data capture), ePRO (electronic patient-reported outcomes)/eCOA (eClinical outcome assessment), imaging, sensors, etc. Selecting and building collections of individual, siloed technologies for each mode of data acquisition leads to inefficiencies and potential inconsistencies in the study build for each system. This approach is resource-intensive, needing IT and clinical programming support to integrate systems, enable and manage data flow, and aggregate the data for holistic review.
Depending on the study, we may need to collect existing patient data from multiple sources (Fig. 1). The number of data sources used within studies is increasing, with some using more than ten.
This leads to a complex ecosystem of applications and integrations. For example: getting EHR (electronic health record) data into the EDC system; linking objective sensor and subjective eCOA data; capturing images through a mobile device and sending them to an imaging review system; and making all patient data visible in one place (the EDC system, for example).
Figure 1. Examples of data sources within a clinical trial.
Once the data has been acquired, how do we achieve complete oversight of data integrity and quality with minimal latency? Our processes are often predicated on traditional site visit data, collected via EDC and based on exhaustive data point-by-data point cleaning. As more complex study design methods are increasingly adopted, an exponential increase in the variety, volume, and velocity of data being collected has necessitated the transformation of data review processes.
Patient Centricity & Data Centricity
To enable a true patient-centric experience, we need to increase patient participation and diversity and reduce any patient burdens to improve patient retention. In parallel, maintaining data quality oversight over all data sources without adding more resources, time, and cost is important. We need data centricity: an approach that looks at data as a whole, from study design and build, through data acquisition and review, to regulatory submission.
Speaking at the Association of Clinical Data Management (ACDM) 2024 conference in Copenhagen, we shared how data centricity helps deliver patient centricity.
Centralized Data Definition That Drives Data Integration and Standardization
Data centricity starts at the study design and build stage, with a centralized approach that drives study build efficiencies, downstream data integration, and standardization.
Imagine taking a protocol and configuring definitions of the data to be collected for each clinical trial activity, creating the outline of a ‘puzzle’ where the empty ‘puzzle slots’ are calibrated to accept specific types of data; then, when the data is acquired, it simply slots into place, automatically aggregated, standardized, and contextualized. This would be agnostic of the data sources; for example, site-captured data, patient-entered information, or sensor-collected data.
An example of this is shown in Figure 2.
Figure 2. Defining the puzzle.
Defining the puzzle starts with digitally creating the schedule of activities from the protocol, defining the data that will be collected from each activity, regardless of how it’s acquired, and then using those data definitions to define the data acquisition experiences (EDC forms, eCOA questionnaires, etc).
We can use a library of common data definitions (called biomedical concepts) and add custom datasets as needed. For example: we have a study that will collect vital signs, physical function assessment, and lab data. Those data definitions can quickly and easily build out data acquisition experiences. In Figure 3, the combined data from multiple sources, such as an electronic case report form (eCRF), a sensor, and eCOA, will provide the physical functions assessments and lab data ingestion that outputs the blood sugar level data.
Figure 3. Example of data output from activity datasets.
Define Once, Build Many Data Acquisition Experiences
Rather than taking the protocol and creating multiple specifications for different data capture systems, this approach defines the schedule of activities and activity data sets, pushing those into the design and implementation of the data capture systems instead. This brings significant efficiencies in study-build timelines and eliminates inconsistencies in data definitions across systems. When there’s a protocol amendment, it also provides a central definition of the study that enables an impact analysis to quickly and easily determine affected areas of the study, including the data capture systems that are potentially impacted by study design changes (Fig 4).
Figure 4. Design once, build many data acquisition experiences.
Downstream Benefits
Creating the puzzle upfront in the study build means that when data (the puzzle pieces) is acquired, it’s automatically slotted into the correct place within the puzzle—the correct dataset (vital signs, physical functions, etc). and its activity context (visits, dates, etc). It’s then possible to automate data standardization as it’s captured, in near real-time, using the data definitions created in advance. This reduces reliance on programming resources to integrate and standardize data, eliminating latency in data availability for review.
Unified Data Quality Oversight Powered by Automation, AI, and Analytics
Without unified, real-time data, data is often out of date by the time the clinical data management and other teams receive it. This can occur when data preparation is done offline and by different teams for different purposes (for example, when data managers are working with different versions of data to what’s used by the central monitors, medical monitors, and the safety team). Add the time it takes to integrate and standardize data ready for review or analysis, and it’s easy to see how problems arise.
While we know that individuals with different functions and roles need to look at data through different lenses, and need specialist tools to perform their tasks, working from the same contemporaneous data is necessary to ensure harmonized delivery of high-quality data and visibility of progress towards that common goal.
Automation, AI, and analytics can achieve holistic data quality oversight while reducing time and effort to perform repetitive and resource-intensive tasks, such as creating listings, writing and posting queries, reconciling common datasets, and building patient profiles.
AI can also shine a light on areas of data that may need attention by data managers and monitors. From a reporting, visualization, and analysis perspective, dashboards can surface critical status information to the relevant stakeholders in a timely fashion. No more generating ad hoc reports at the end of the day because your executives want to know what progress has been made towards achieving clean data and database lock.
Figure 5. Integrated, real-time data for all stakeholders.
To achieve this, the ideal platform (Fig. 5) needs to support four key areas: data acquisition, data ingestion and management, data transformation and standardization, and data monitoring and delivery. The Medidata Platform’s advanced data architecture enables the foundations for the Platform to achieve all of this with Medidata Clinical Data Studio, including:
- Centralizing study design and build processes
- Acquiring data from multiple sources
- Creating any dataset you need for review and monitoring tasks, or transforming data into standard formats
- Providing advanced data quality experiences for all stakeholders
- Leveraging the unified, standardized data
- Creating datasets in a standard format for study analysis and defining new data products
- Supporting open access to your data at any point in its lifecycle
In Summary
Working with centralized, unified, real-time data within our powerful data and analytics platform empowers every stakeholder; it enables data tasks to be performed in parallel, automates repetitive tasks, surfaces issues faster, and reduces time to clean data and database lock.
A data-centric clinical research platform—where data centricity runs from study design and build through to submission-ready data—is a key enabler in delivering patient centricity in terms of flexibility in how patients participate in clinical trials and data is collected. This provides optimized data acquisition experiences, letting research teams gain greater patient insights and detect and take action on patient safety signals quickly.
After all, patient centricity needs data centricity.
Learn more about Medidata’s Clinical Data Management capabilities.
Enjoyed this article? Click here to share it with your network.
Contact Us
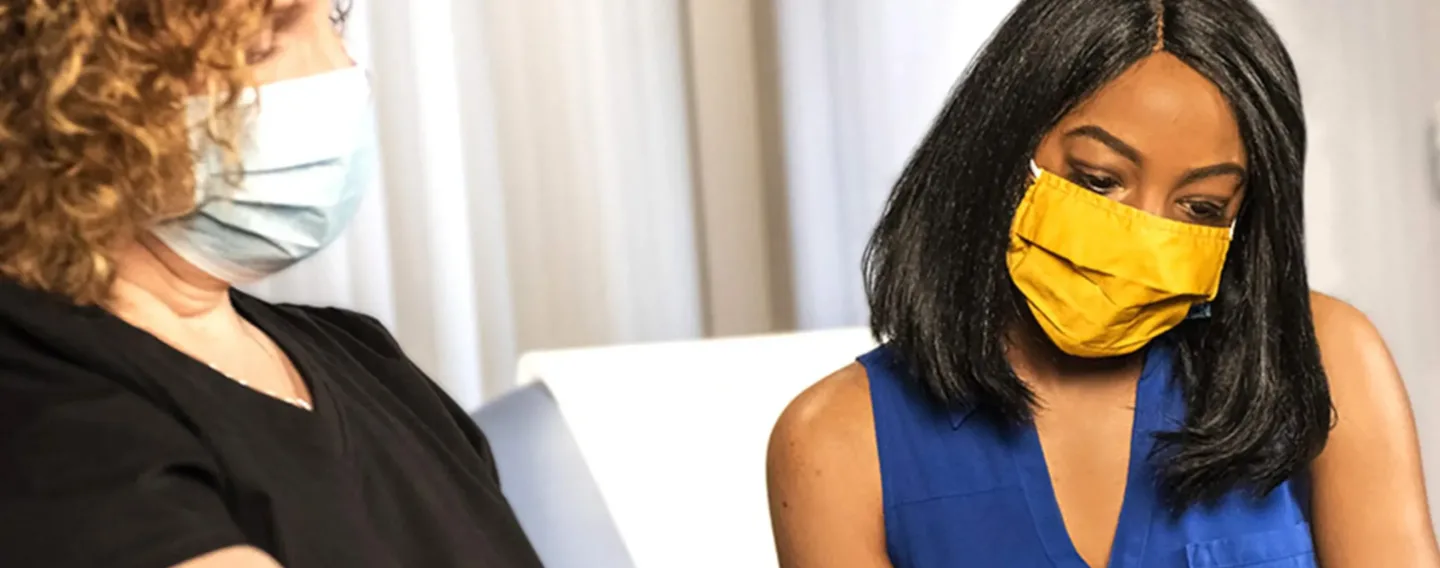