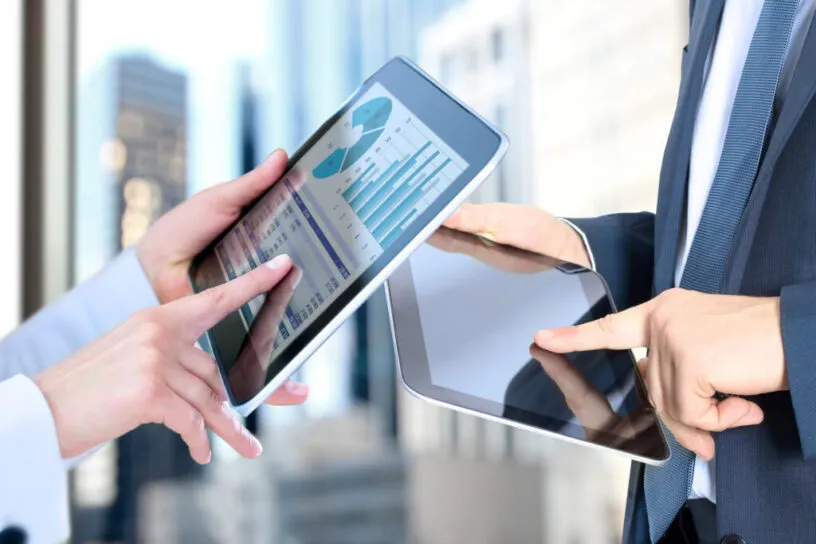
Marketing mix models are leveraged to assess the impact of multiple promotional channels within a company or brand on their return on investment or ROI. Bayesian regressions combined with nonlinear transformations and ad stock decay are the common marketing data models of choice. But as the data landscape rapidly shifts with technology-driven advances such as AI and automation, the ability to choose the best algorithm in real-time grows more challenging.
Hartaig Singh and Vikas Hiremath of Medidata Solutions, a Dassault Systèmes Company, recently discussed how to tackle this issue. They conducted an analysis to test the efficiency of AI/machine learning (ML) models over traditional Bayesian regressions and identified some interesting advantages that may disrupt the preferred industry standard. AI/ML models require significantly fewer assumptions, parameters, and less prior information—drastically reducing time required and modeler discretion. In addition, AI/ML model feature importance may be correlated with the quality of response curve fit, which is a leading indicator for response curve accuracy.
Based on a simulated data set with segments, Medidata fit one AI/ML model and four different Bayesians regressions to assess the impacts of three promotional media channels on monthly ROI. C- and s-shaped response curves were plotted using specified hill parameters for simulated healthcare provider specialties/segments. As shown in the Figure below, true responses were compared with model estimates to see if the curve shape was being estimated well.
The AI/ML model captured segment-level responses and true fits significantly better than their Bayesian model counterparts. By design, AI/ML models are better than Bayesian models at handling a larger number of features and collinearity, capturing non-linearity in response curves, more accurately modeling real behavior, and identifying the interplay between features without having to specify this in explicit detail. Not only does this remove the need to manually define forced saturation transformations, but it also minimizes the danger of human/user error and the potential for bias in marketing data models.
Digital response on hematologists (A) and oncologists (B):
True responses were compared with model estimates. Responses were normalized to standardize scale.
As Hartaig noted, “In the past, the industry standard was to use Bayesian methods, but it is a huge ask because parameters must be set, there are more specifications such as defining saturation, it is up to the modeler’s skill versus data-driven, and so on,” meaning Bayesian models require precious time and resources to properly fit a marketing data model and generate any insights. But the non-linearity of an AI/ML model solves most of this. These advantages aid in reducing model complexity and modeler discretion, enabling more granular level insight, scalability, and automation.
With these insights in mind, advanced predictive models capable of solving complex marketing problems are possible. Bayesian is still probably the best case when the manual effort and exhaustive pre-work has been completed, but who really has time for that in the digital age?
For more details, check out the study poster. And learn more about Commercial Analytics.
Contact Us
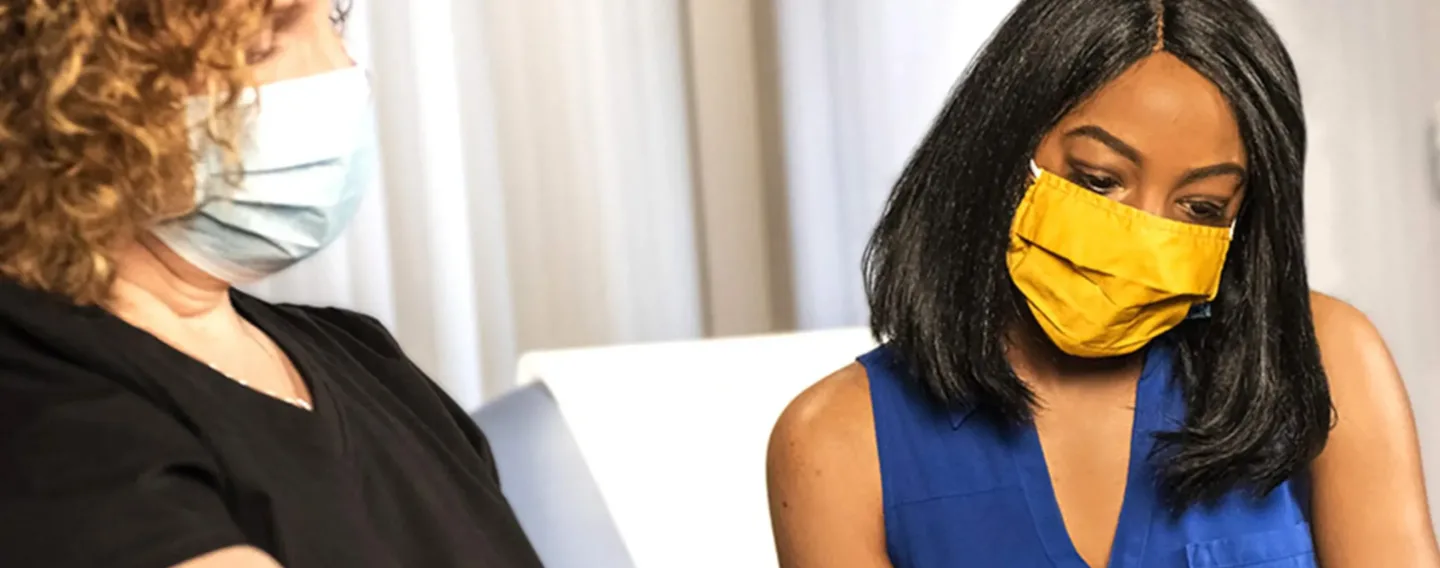