Next Generation of Clinical Monitoring | Part 1: Detect Variability in Adverse Event Reporting
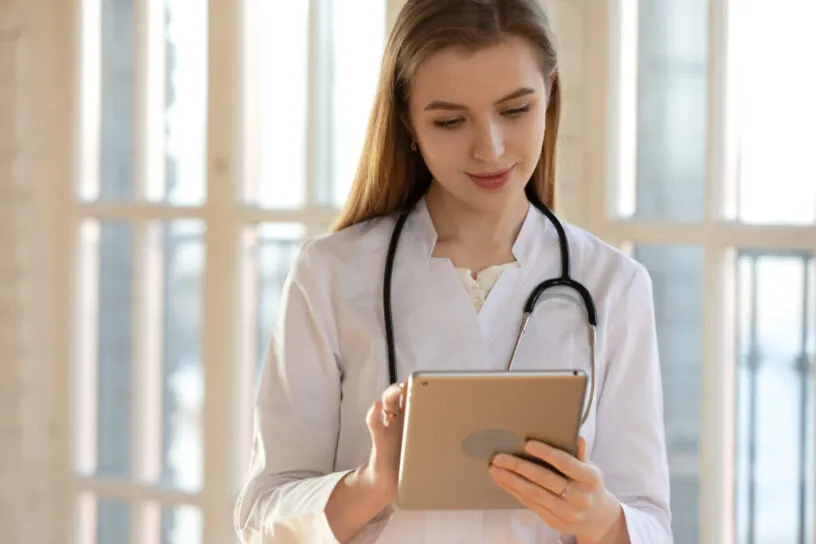
Modern clinical trials continue to increase in complexity as they include more decentralized elements, adaptive study designs, and data originating from multiple sources. Comprehensive data and risk surveillance technologies are now addressing this complexity, helping study teams ensure data veracity and quality across large datasets and supplementing traditional site-based monitoring activities.
Conventional monitoring methods—typically involving thorough site audits and monitoring activities via manual source data review (SDR) and source data verification (SDV)—are both inefficient and difficult to scale across global programs. These methods provide a limited picture of overall data quality and trial risk, as site data is reviewed in isolation. When it comes to early detection of study-level data risks, such as variability in adverse event (AE) reporting, site-based monitoring approaches alone are insufficient.
Why Accurate Adverse Event Detection Matters
Variability in AE reporting is a recurring problem in clinical trials, especially for multicenter and global studies. Studies have shown that AE reporting rates vary based on the country for a number of reasons: variation in understanding of protocol and clinical research requirements, cultural differences resulting in not reporting milder events, and perceived stigmas around reporting adverse events.
Overall, variability in AE reporting can negatively impact patient safety and clinical trial data integrity. Under-reporting can delay the identification of a significant new risk to patient safety and impact the accuracy and completeness of drug safety profiles. Over-reporting can introduce noise into datasets by confounding diagnoses with signs and symptoms. This increases the need for unnecessary data review and delays the detection of safety risks. Accurate, complete, and on-time reporting of AEs is essential to evaluate and manage a study's safety data, and impacts critical decisions during the trial.
Centralized Statistical Monitoring of AE Reporting
Analytics and statistical methodologies can be used to objectively identify reporting pattern differences across safety domains. These analyses identify data outliers across the study and let users—central monitors, data managers, or anyone in charge of safety monitoring—focus on a subset of trial sites to investigate further before generating an issue for an on-site or remote monitor. This highly contextual vantage point allows earlier insight to signals of risk, and encourages better allocation of monitoring resources. Statistically significant signals of AE reporting variation can then be flagged to clinical trial monitors as issues to further investigate the root cause, trigger site re-education, and make sure the trial site is equipped to collect accurate and timely safety information.
As part of the evaluation, the following data interrogation criteria should be considered:
Criteria | Consideration |
Number of Patients, Events, and Overall Drug Exposure | Consider dense enrollment and all patients being in the early stages of the trial. (See Figure 1) |
Reporting Pattern of Concomitant Medications (Conmeds) | Does the clinical trial site have significantly more concomitant medications than what we see with other sites? Do we see concomitant medications without associated AEs and medical histories? |
Medical History | Are there too many or too few? Are there healthier patients with fewer events or missing information? |
Reporting Patterns | Do we see any patterns related to the type of underreported events, e.g., mild events? |
Patient Populations | Evaluation of information in the context of impacted patients or subpopulation of patients. (See Figure 2) |
Data Currency | Is the adverse event reporting variance due to a backlog of information not being entered? Evaluate in particular the AE Entry Delay KRI. (See Figure 3) |
Fig 1: AE Under-Over Reporting
Fig 2: Patient Profiles – Safety Timeline Tables
Fig 3: KRI Dashboard: AE Entry Delay Metric
Summary
Traditional clinical trial monitoring processes do not provide visibility into reporting patterns where cross-site evaluation is needed to identify potential risk signals like AE underreporting. Leveraging data and surveillance technologies with advanced analytics, intuitive visualizations, and real-time data from all patient data sources ensures adequate data and risk surveillance, as well as comprehensive and timely safety reporting.
Holistic data surveillance requires all data available in one place; this can be achieved on a secure, stable, and scalable cloud-based unified platform, such as the Medidata Clinical Cloud®, on which trial data from almost any source can be aggregated in one place for analysis. Medidata Detect, which is powered by data aggregated from the Medidata platform, is an end-to-end data surveillance and centralized monitoring solution that lets both data management and clinical operations teams monitor and limit risks to patient safety and data integrity. This facilitates aggregation of safety information from eCRF and non-eCRF sources—data coming not only from the AE data set, but all surrounding data that is collecting safety information—and should be cross checked as part of AE reporting evaluation, including lab data, patient-reported outcomes, sensor data, and imaging.
Download our white paper to learn more about modernizing clinical trial oversight:
Explore Related Articles
Contact Us
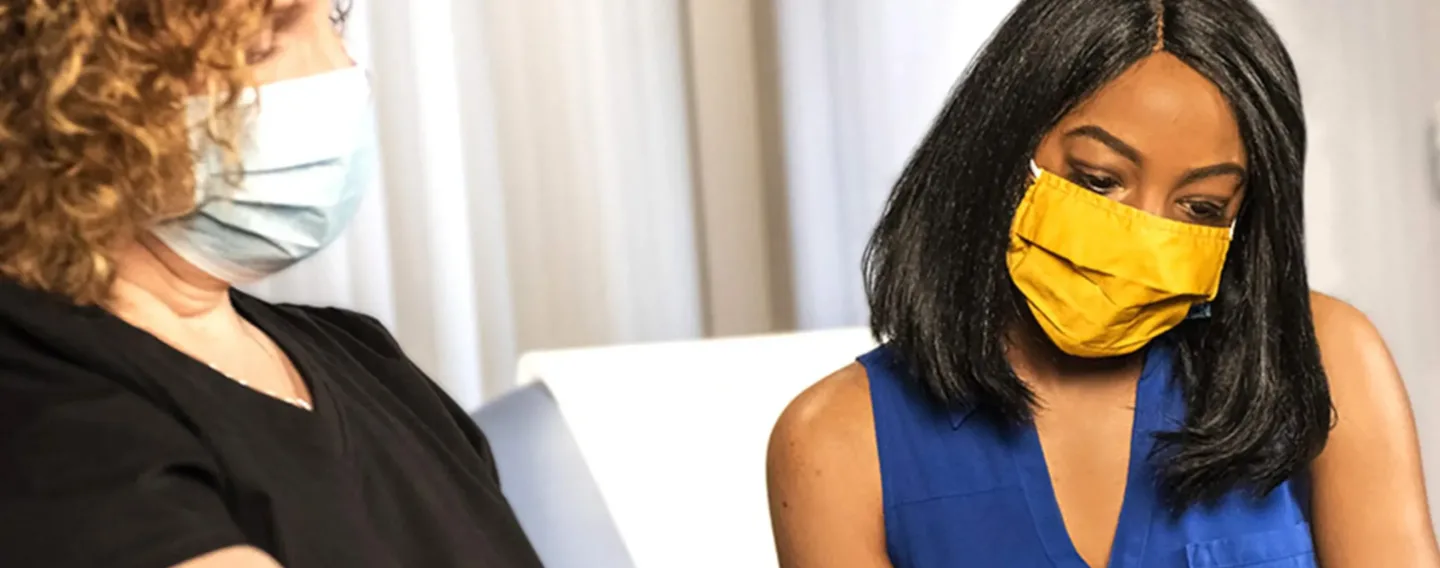