No More Bottlenecks: How to Reimagine Workflows to Get Faster Database Lock in Clinical Trials
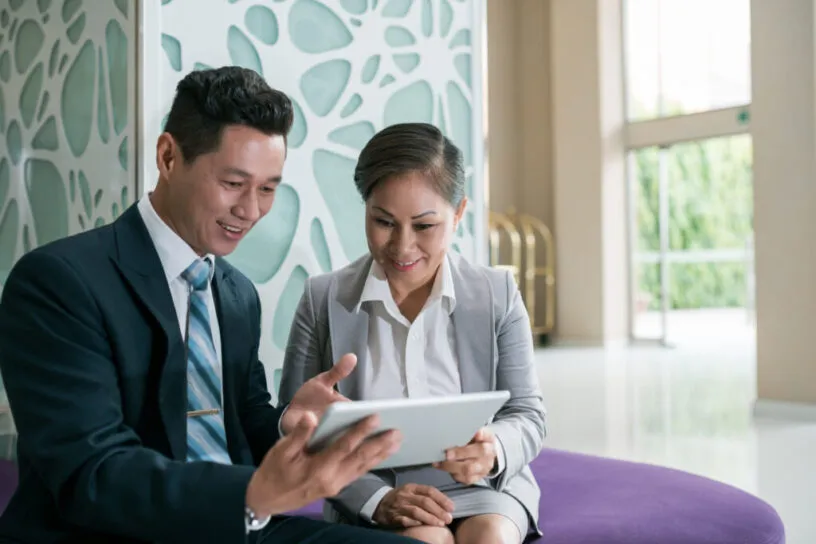
High-quality clinical data analyzed promptly is essential for bringing lifesaving drugs to market faster. Several steps in the clinical trial workflow must be orchestrated seamlessly as per the study protocol and regulatory requirements. Any data recorded during the study must be collated into a database where it can be analyzed. All database entries must be free of errors or discrepancies, cleaned up if required, reviewed, and finalized before analysis. Once a database has been finalized, it is set to be locked, meaning no further changes are permitted. Reaching database lock early is important to avoid any delays in study completion and analysis of the clinical trial results.
What Are the Key Challenges in the Steps Leading to Clinical Trial Database Lock?
Clinical Data Entry
Clinical trial data can be captured through various methods, including paper-based forms, electronic systems such as electronic health records (EHRs) or electronic medical records (EMRs), lab or image reports, and other disconnected electronic sources outside the electronic data capture (EDC) system. Whether you are transcribing data from paper and manually entering it into the EDC or using swivel chair integration of electronic records—viewing and comparing two computer screens and transferring the data from one system to the other—the challenge is to make sure that data has been entered accurately with a minimum of repetition. Such data entry methods are slow, prone to error, and riddled with repetitive processes. As clinical data management has evolved to be more complex, today’s EDC systems must also evolve to meet the current challenges.
To maximize efficiency in data entry, electronic case report forms (eCRFs) must be designed clearly so that trial sites know what data must be captured in the EDC system. Plus, the EDC system must be able to support dynamic data capture: it must have the ability to present relevant questions based on information that the sites entered into their electronic forms. For example, if a site responds to a question about the patient’s gender by identifying the patient as female, then it may be appropriate to ask whether the woman might be pregnant if that information is relevant to the study. Based on the response to the pregnancy question, other relevant parts of the form must become visible to the site.
Moreover, the system must be able to record and save the response to each question at the time it is entered before moving on to the next question rather than waiting until the entire form has been filled out to record all the entered data. Systems that do not offer this dynamic feature are likely to raise unnecessary queries.
Source Data Verification (SDV)
In this step of the clinical data management and monitoring process, source data from paper documents or other systems are verified to make sure that the information entered into the EDC completely matches the original. This process is very time-consuming (particularly if 100% SDV is performed) and creates a bottleneck in the clinical workflow.
Data Aggregation and Reconciliation in Clinical Data Management
The next challenge is bringing together patient data from multiple systems, such as eCRF data, electronic clinical outcome assessments (eCOAs), electronic patient-reported outcomes (ePROs), sensors, wearable devices, lab test results, imaging reports, etc., into a holistic, consolidated view. In conventional systems, this step involves programmers extracting and joining data from the siloed systems. This joined data must be cross-checked to confirm that all data entered for a particular patient in one system exactly matches the same patient’s information recorded in another system.
Lack of interoperability between siloed systems and different electronic sources is often an issue that disrupts the seamless integration of data. Once data aggregation is complete, it has to be reconciled before proceeding to data review and cleaning. Reconciliation is done to verify that the transfer of data has been complete and records are not missing, erroneous, repeated, out of format, or referenced to the wrong tables or systems. To keep up with the rapid advancements in the clinical trial workflow, a unified clinical research platform should be used—one that is capable of capturing and aggregating data from multiple sources, thereby drastically reducing or even eliminating the need for programming resources to aggregate and reconcile clinical data.
Data Review and Cleaning in Clinical Trials
The goal of this step is to check the data quality and integrity after the data has been extracted and aggregated. This process is complicated by the fact that the data listings involved have often been created outside the core clinical systems, requiring spreadsheets to be used to keep track of what has been reviewed and cleaned—a method that is exhaustive, inefficient, and ineffective. There are alternative clinical data repository-type tools available, but they still require manual intervention to extract, manipulate, and upload data from multiple systems into the clinical data repository, making this process burdensome and slowing the time to database lock.
Since accuracy is of paramount importance in clinical trials, a traditional review process requires that all data points in a trial be checked, queried in case of any discrepancy, and resolved. But with the recent technological advancements in data capture and the shift to decentralized clinical trial designs, a large clinical trial can generate a tidal wave of information with over 3 million data points.
Studies have shown that on average, only 2-4% of trial data end up requiring change after capture, illustrating the tedious and time-consuming inefficiencies inherent in the traditional approaches that try to query and clean 100% of the data. Query-based data cleaning approaches are hence turning out to be unnecessary and ineffective as data acquisition methods have become much more sophisticated and less prone to error in the past 15 years. There is a need for better strategies for faster and more effective reviewing and cleaning of the data, rather than checking everything.
A unified platform such as the Medidata Clinical Cloud® provides a holistic view of clinical trial data coming from different sources (eCRF, imaging data, sensor data, etc.). The platform also brings together patient profile data from those multiple sources without the need for complex programming steps. Data managers simply drag-and-drop the datasets of interest and create an aggregated view of the labels, lists, or tables for review, rather than requiring specialist programming resources. The patient profile includes links back to the source data. For example, if there was an issue with a data point in the patient profile that comes from an electronic case report form field, it can be easily traced back to the EDC system, and a query raised from the patient profile that is visible to the relevant site within the EDC system.
Data Transformation
Cleaned clinical trial data must be transformed into a standard format before analysis because raw data is captured from multiple systems, which could follow different semantics or data derivation rules. Automated data transformations using artificial intelligence- (AI) and machine learning- (ML) based approaches can achieve data interoperability faster when handling data with different standards and naming conventions. Data transformations that are done in real-time rather than in a batch mode or after study completion drastically reduce the time required to reach database lock, accelerating the entire clinical study workflow.
How Does the Medidata Clinical Cloud® Get to Database Lock Faster?
The Medidata Clinical Cloud has advanced capabilities that streamline clinical trial workflow and achieve database lock faster. Its capabilities are built to overcome the hurdles at each step of the data management process:
Data Entry
Medidata Rave EDC lets sites enter data quickly and easily into dynamic eCRFs. eConsent and eCOA/ePRO data captured via myMedidata are automatically available in Rave EDC. Relevant imaging data is also available through interoperability with Rave Imaging, and summarized sensor data comes in from Medidata’s Sensor Cloud. This provides a consolidated overview of patient data for sites and sponsors/CROs.
Wherever possible, Medidata minimizes the need for duplicate data entry. Via Rave Safety Gateway, adverse event data entered into Rave EDC is automatically transferred as a safety case to the sponsor’s safety system. And sites can randomize a patient and dispense medication directly from Rave EDC via its unification with Rave RTSM (randomization and trial supply management), eliminating the need to log into and enter data in a separate system.
Data Aggregation and Reconciliation
With Patient Profiles in Medidata Detect, patient data from multiple sources can be aggregated into a single dataset. Medidata Detect obviates the need for complex programming, with Patient Profiles created quickly using simple drag-and-drop and layering events onto the study timeline. Patient Profiles can be visualized in multiple ways, enabling monitors and data managers to more easily spot data issues.
Data Review and Cleaning
Conventional data review approaches require data listings and external trackers such as spreadsheets. In contrast, Medidata Detect’s Patient Profiles application has Data Reviewer capabilities built-in. In addition to the eCRF query functionality within Rave EDC, Medidata Detect supports the raising of queries that are directed to the relevant site via Rave EDC, providing one place and unified mechanisms for sites to respond to potential data issues. Medidata Detect is also a proactive risk-minimization tool that allows automated, intelligent detection of patterns, trends, anomalies, and outliers, enabling issues in large volumes of data from multiple sources to be surfaced quickly. This drastically improves data quality and integrity while reducing data correction and database lock cycle times.
Data Transformation
Medidata is evolving the way that data is transformed from manual batch processing to automated, streamed transmission of submission-ready output. One example is how AI and ML are being applied to improve the efficiency of image assessments, which can have a lot of variability and significant differences in semantic classifications. Using multiple applications on the Medidata Clinical Cloud, customers have achieved database lock sooner (by 9 days)1, and have shortened their studies (by 2 months)2.
Contact us today to discuss how Medidata can help you reimagine your clinical trial workflows and accelerate clinical development.
1Analysis of difference in median LPLV to DBL time for EDC + at least one additional product vs EDC only studies from 2017 to 2021.
2Analysis of difference in median FPI to LPLV time for EDC + at least one additional product vs. EDC only studies (p<0.05) 2017 to 2021; Reduction of 59 Days.
Explore Related Articles
Contact Us
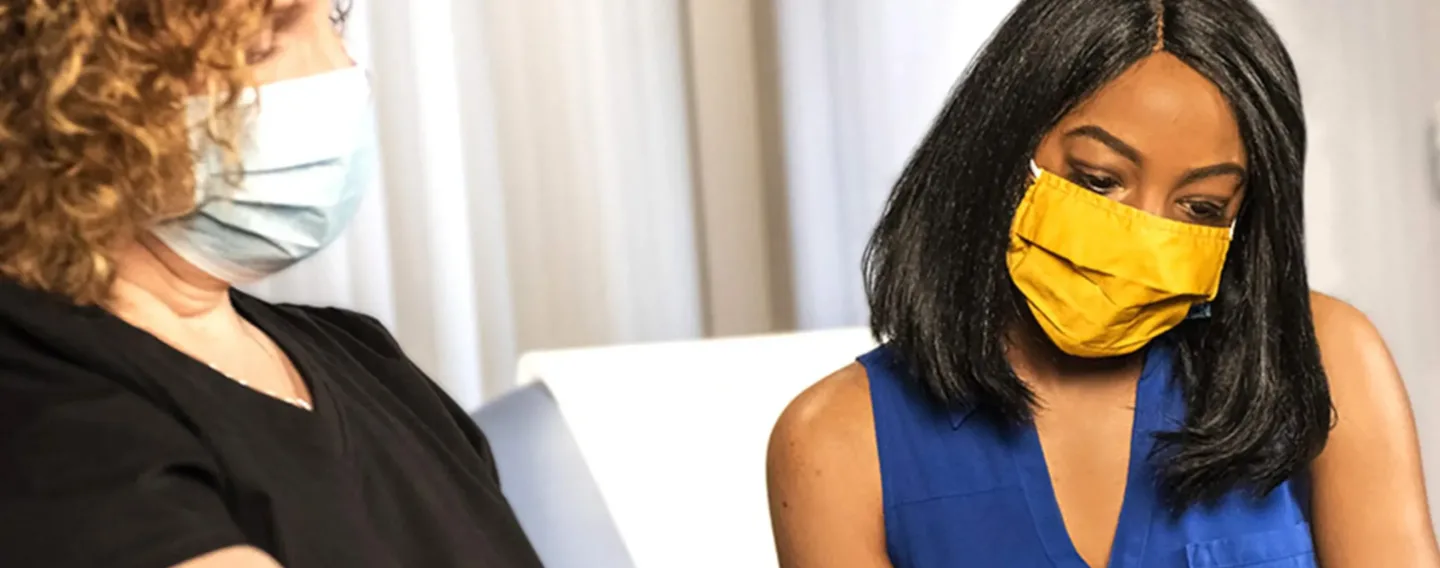